ๅ็ ง(Reference) : ใใใผใฟใตใคใจใณใใฃในใๅไผในใญใซๅฎ็พฉๅงๅกใใฎใใใผใฟใตใคใจใณใน100ๆฌใใใฏ๏ผๆง้ ๅใใผใฟๅ ๅทฅ็ทจ๏ผใ
The Data Scientist Society Github :
Data Science 100 Knocks (Structured Data Processing) URL :
-
Note: This is an ipynb file originally created by The Data Scientist Society(ใใผใฟใตใคใจใณใใฃในใๅไผในใญใซๅฎ็พฉๅงๅก) and translated from Japanese to English by DeepL.
-
The reason I updated this file is to spread this practice, which is useful for everyone who wants to practice Python, from beginners to advanced engineers.
-
Since this data was created for Japanese, you may face language problems when practicing. But do not worry, it will not affect much.
Getting Started
- First, run the following cells
- Import the necessary libraries and read data from the database (PostgreSQL)
- The following cell imports libraries that are expected to be used, such as pandas.
- If there are other libraries you wish to use, install them as needed (you can also install them by โ!pip install library nameโ).
- The process can be divided into multiple times.
- Names, addresses, etc. are dummy data and are not real.
import os
import pandas as pd
import numpy as np
from datetime import datetime, date
from dateutil.relativedelta import relativedelta
import math
import psycopg2
from sqlalchemy import create_engine
from sklearn import preprocessing
from sklearn.impute import SimpleImputer
from sklearn.model_selection import train_test_split
from sklearn.model_selection import TimeSeriesSplit
from imblearn.under_sampling import RandomUnderSampler
if 'PG_PORT' in os.environ:
pgconfig = {
'host': 'db',
'port': os.environ['PG_PORT'],
'database': os.environ['PG_DATABASE'],
'user': os.environ['PG_USER'],
'password': os.environ['PG_PASSWORD'],
}
# Connector for pd.read_sql
conn = psycopg2.connect(**pgconfig)
df_customer = pd.read_sql(sql='select * from customer', con=conn)
df_category = pd.read_sql(sql='select * from category', con=conn)
df_product = pd.read_sql(sql='select * from product', con=conn)
df_receipt = pd.read_sql(sql='select * from receipt', con=conn)
df_store = pd.read_sql(sql='select * from store', con=conn)
df_geocode = pd.read_sql(sql='select * from geocode', con=conn)
else:
if not os.path.exists('data/'):
!git clone https://github.com/The-Japan-DataScientist-Society/100knocks-preprocess
os.chdir('100knocks-preprocess/docker/work/')
dtype = {
'customer_id': str,
'gender_cd': str,
'postal_cd': str,
'application_store_cd': str,
'status_cd': str,
'category_major_cd': str,
'category_medium_cd': str,
'category_small_cd': str,
'product_cd': str,
'store_cd': str,
'prefecture_cd': str,
'tel_no': str,
'postal_cd': str,
'street': str
}
df_customer = pd.read_csv("data/customer.csv", dtype=dtype)
df_category = pd.read_csv("data/category.csv", dtype=dtype)
df_product = pd.read_csv("data/product.csv", dtype=dtype)
df_receipt = pd.read_csv("data/receipt.csv", dtype=dtype)
df_store = pd.read_csv("data/store.csv", dtype=dtype)
df_geocode = pd.read_csv("data/geocode.csv", dtype=dtype)
fatal: destination path '100knocks-preprocess' already exists and is not an empty directory.
Exercise
P-001: Display the first 10 items of all items from the receipt details data (df_receipt) and visually check what data is held.
df_receipt.head(10)
sales_ymd | sales_epoch | store_cd | receipt_no | receipt_sub_no | customer_id | product_cd | quantity | amount | |
---|---|---|---|---|---|---|---|---|---|
0 | 20181103 | 1541203200 | S14006 | 112 | 1 | CS006214000001 | P070305012 | 1 | 158 |
1 | 20181118 | 1542499200 | S13008 | 1132 | 2 | CS008415000097 | P070701017 | 1 | 81 |
2 | 20170712 | 1499817600 | S14028 | 1102 | 1 | CS028414000014 | P060101005 | 1 | 170 |
3 | 20190205 | 1549324800 | S14042 | 1132 | 1 | ZZ000000000000 | P050301001 | 1 | 25 |
4 | 20180821 | 1534809600 | S14025 | 1102 | 2 | CS025415000050 | P060102007 | 1 | 90 |
5 | 20190605 | 1559692800 | S13003 | 1112 | 1 | CS003515000195 | P050102002 | 1 | 138 |
6 | 20181205 | 1543968000 | S14024 | 1102 | 2 | CS024514000042 | P080101005 | 1 | 30 |
7 | 20190922 | 1569110400 | S14040 | 1102 | 1 | CS040415000178 | P070501004 | 1 | 128 |
8 | 20170504 | 1493856000 | S13020 | 1112 | 2 | ZZ000000000000 | P071302010 | 1 | 770 |
9 | 20191010 | 1570665600 | S14027 | 1102 | 1 | CS027514000015 | P071101003 | 1 | 680 |
Commentary :
The codedf_receipt.head(10)
is used to display the first 10 rows of a Pandas DataFrame calleddf_receipt
.
In Pandas, a DataFrame is a two-dimensional labeled data structure with columns of potentially different data types. It is similar to a spreadsheet or SQL table.
The.head()
method in Pandas is used to display the first n rows of a DataFrame. By default, it displays the first 5 rows. In this case,.head(10)
is used to display the first 10 rows of the DataFrame.
P-002: Display 10 items from the receipt details data (df_receipt), specifying the columns in the order of sales_date (sales_ymd), customer_id (customer_id), product_code (product_cd) and sales amount (amount).
df_receipt[['sales_ymd', 'customer_id', 'product_cd', 'amount']].head(10)
sales_ymd | customer_id | product_cd | amount | |
---|---|---|---|---|
0 | 20181103 | CS006214000001 | P070305012 | 158 |
1 | 20181118 | CS008415000097 | P070701017 | 81 |
2 | 20170712 | CS028414000014 | P060101005 | 170 |
3 | 20190205 | ZZ000000000000 | P050301001 | 25 |
4 | 20180821 | CS025415000050 | P060102007 | 90 |
5 | 20190605 | CS003515000195 | P050102002 | 138 |
6 | 20181205 | CS024514000042 | P080101005 | 30 |
7 | 20190922 | CS040415000178 | P070501004 | 128 |
8 | 20170504 | ZZ000000000000 | P071302010 | 770 |
9 | 20191010 | CS027514000015 | P071101003 | 680 |
Commentary :
The codedf_receipt[['sales_ymd', 'customer_id', 'product_cd', 'amount']].head(10)
is used to display the first 10 rows of a subset of columns from a Pandas DataFrame calleddf_receipt
.
The codedf_receipt[['sales_ymd', 'customer_id', 'product_cd', 'amount']]
is used to select a subset of columns from the DataFramedf_receipt
. Specifically, it selects the columns namedsales_ymd
,customer_id
,product_cd
, andamount
.
The resulting DataFrame contains only these selected columns. The.head(10)
method is then used to display the first 10 rows of this subset DataFrame.
P-003: From the receipt details data (df_receipt), specify the columns in order of sales_date (sales_ymd), customer_id (customer_id), product_code (product_cd) and sales_amount (amount) and display 10 items. However, extract while renaming the items from sales_ymd to sales_date.
df_receipt[['sales_ymd', 'customer_id', 'product_cd', 'amount']]. \
rename(columns={'sales_ymd': 'sales_date'}).head(10)
sales_date | customer_id | product_cd | amount | |
---|---|---|---|---|
0 | 20181103 | CS006214000001 | P070305012 | 158 |
1 | 20181118 | CS008415000097 | P070701017 | 81 |
2 | 20170712 | CS028414000014 | P060101005 | 170 |
3 | 20190205 | ZZ000000000000 | P050301001 | 25 |
4 | 20180821 | CS025415000050 | P060102007 | 90 |
5 | 20190605 | CS003515000195 | P050102002 | 138 |
6 | 20181205 | CS024514000042 | P080101005 | 30 |
7 | 20190922 | CS040415000178 | P070501004 | 128 |
8 | 20170504 | ZZ000000000000 | P071302010 | 770 |
9 | 20191010 | CS027514000015 | P071101003 | 680 |
Commentary :
The codedf_receipt[['sales_ymd', 'customer_id', 'product_cd', 'amount']].rename(columns={'sales_ymd': 'sales_date'}).head(10)
is used to select a subset of columns from a Pandas DataFrame calleddf_receipt
, rename one of the columns, and then display the first 10 rows of the resulting DataFrame.
The codedf_receipt[['sales_ymd', 'customer_id', 'product_cd', 'amount']]
is used to select a subset of columns from the DataFramedf_receipt
. Specifically, it selects the columns namedsales_ymd
,customer_id
,product_cd
, andamount
.
The.rename()
method is then used to rename thesales_ymd
column tosales_date
. This method returns a new DataFrame with the renamed column. The argumentcolumns={'sales_ymd': 'sales_date'}
specifies a dictionary that maps the old column name to the new column name.
Finally, the.head(10)
method is used to display the first 10 rows of the resulting DataFrame.
P-004: From receipt details data (df_receipt), specify columns in the order of sales_date (sales_ymd), customer ID (customer_id), product code (product_cd) and sales amount (amount) and extract data satisfying the following conditions.
- Customer ID (customer_id) is โCS018205000001โ
# Code example 1 (when using query)
df_receipt[['sales_ymd', 'customer_id', 'product_cd', 'amount']]. \
query('customer_id == "CS018205000001"')
sales_ymd | customer_id | product_cd | amount | |
---|---|---|---|---|
36 | 20180911 | CS018205000001 | P071401012 | 2200 |
9843 | 20180414 | CS018205000001 | P060104007 | 600 |
21110 | 20170614 | CS018205000001 | P050206001 | 990 |
27673 | 20170614 | CS018205000001 | P060702015 | 108 |
27840 | 20190216 | CS018205000001 | P071005024 | 102 |
28757 | 20180414 | CS018205000001 | P071101002 | 278 |
39256 | 20190226 | CS018205000001 | P070902035 | 168 |
58121 | 20190924 | CS018205000001 | P060805001 | 495 |
68117 | 20190226 | CS018205000001 | P071401020 | 2200 |
72254 | 20180911 | CS018205000001 | P071401005 | 1100 |
88508 | 20190216 | CS018205000001 | P040101002 | 218 |
91525 | 20190924 | CS018205000001 | P091503001 | 280 |
Commentary :
The codedf_receipt[['sales_ymd', 'customer_id', 'product_cd', 'amount']].query('customer_id == "CS018205000001"')
is used to select a subset of rows from a Pandas DataFrame calleddf_receipt
, where thecustomer_id
column is equal to a specific value (CS018205000001
).
The codedf_receipt[['sales_ymd', 'customer_id', 'product_cd', 'amount']]
is used to select a subset of columns from the DataFramedf_receipt
. Specifically, it selects the columns namedsales_ymd
,customer_id
,product_cd
, andamount
.
The.query()
method is then used to filter the rows of the DataFrame based on a specific condition. In this case, the condition is that thecustomer_id
column must be equal to the value"CS018205000001"
. The backslash (\
) at the end of the first line indicates that the code is continued on the next line for better readability.
The resulting DataFrame contains only the rows where thecustomer_id
column matches the specified value.
# Code example 1 (without query)
df = df_receipt[['sales_ymd', 'customer_id', 'product_cd', 'amount']]
df[df['customer_id'] == 'CS018205000001']
sales_ymd | customer_id | product_cd | amount | |
---|---|---|---|---|
36 | 20180911 | CS018205000001 | P071401012 | 2200 |
9843 | 20180414 | CS018205000001 | P060104007 | 600 |
21110 | 20170614 | CS018205000001 | P050206001 | 990 |
27673 | 20170614 | CS018205000001 | P060702015 | 108 |
27840 | 20190216 | CS018205000001 | P071005024 | 102 |
28757 | 20180414 | CS018205000001 | P071101002 | 278 |
39256 | 20190226 | CS018205000001 | P070902035 | 168 |
58121 | 20190924 | CS018205000001 | P060805001 | 495 |
68117 | 20190226 | CS018205000001 | P071401020 | 2200 |
72254 | 20180911 | CS018205000001 | P071401005 | 1100 |
88508 | 20190216 | CS018205000001 | P040101002 | 218 |
91525 | 20190924 | CS018205000001 | P091503001 | 280 |
Commentary :
The codedf_receipt[['sales_ymd', 'customer_id', 'product_cd', 'amount']].query('customer_id == "CS018205000001"')
is used to select a subset of rows from a Pandas DataFrame calleddf_receipt
, where thecustomer_id
column is equal to a specific value (CS018205000001
).
The codedf_receipt[['sales_ymd', 'customer_id', 'product_cd', 'amount']]
is used to select a subset of columns from the DataFramedf_receipt
. Specifically, it selects the columns namedsales_ymd
,customer_id
,product_cd
, andamount
.
The.query()
method is then used to filter the rows of the DataFrame based on a specific condition. In this case, the condition is that thecustomer_id
column must be equal to the value"CS018205000001"
. The backslash (\
) at the end of the first line indicates that the code is continued on the next line for better readability.
The resulting DataFrame contains only the rows where thecustomer_id
column matches the specified value.
P-005: Extract data from receipt details data (df_receipt), specifying columns in the order of sales_date (sales_ymd), customer ID (customer_id), product code (product_cd) and sales amount (amount) and satisfying all the following conditions.
- Customer ID (customer_id) is โCS018205000001โ.
- Sales amount (amount) is 1,000 or more.
df_receipt[['sales_ymd', 'customer_id', 'product_cd', 'amount']] \
.query('customer_id == "CS018205000001" & amount >= 1000')
sales_ymd | customer_id | product_cd | amount | |
---|---|---|---|---|
36 | 20180911 | CS018205000001 | P071401012 | 2200 |
68117 | 20190226 | CS018205000001 | P071401020 | 2200 |
72254 | 20180911 | CS018205000001 | P071401005 | 1100 |
Commentary :
The codedf_receipt[['sales_ymd', 'customer_id', 'product_cd', 'amount']] \ .query('customer_id == "CS018205000001" & amount >= 1000')
is used to select a subset of rows from a Pandas DataFrame calleddf_receipt
, where thecustomer_id
column is equal to a specific value (CS018205000001
) and theamount
column is greater than or equal to 1000.
The codedf_receipt[['sales_ymd', 'customer_id', 'product_cd', 'amount']]
is used to select a subset of columns from the DataFramedf_receipt
. Specifically, it selects the columns namedsales_ymd
,customer_id
,product_cd
, andamount
.
The.query()
method is then used to filter the rows of the DataFrame based on multiple conditions. The&
symbol is used to indicate a logical AND operation between the two conditions. The first condition is that thecustomer_id
column must be equal to the value"CS018205000001"
. The second condition is that theamount
column must be greater than or equal to 1000.
The resulting DataFrame contains only the rows where both conditions are satisfied.
P-006: Extract data from receipt details data (df_receipt), specifying the columns in the order of sales_date (sales_ymd), customer ID (customer_id), product code (product_cd), sales quantity (quantity) and sales amount (amount) and satisfying all the following conditions.
- Customer ID (customer_id) is โCS018205000001โ.
- Sales amount (amount) is 1,000 or more or sales quantity (quantity) is 5 or more.
df_receipt[['sales_ymd', 'customer_id', 'product_cd', 'quantity', 'amount']].\
query('customer_id == "CS018205000001" & (amount >= 1000 | quantity >=5)')
sales_ymd | customer_id | product_cd | quantity | amount | |
---|---|---|---|---|---|
36 | 20180911 | CS018205000001 | P071401012 | 1 | 2200 |
9843 | 20180414 | CS018205000001 | P060104007 | 6 | 600 |
21110 | 20170614 | CS018205000001 | P050206001 | 5 | 990 |
68117 | 20190226 | CS018205000001 | P071401020 | 1 | 2200 |
72254 | 20180911 | CS018205000001 | P071401005 | 1 | 1100 |
Commentary :
The codedf_receipt[['sales_ymd', 'customer_id', 'product_cd', 'quantity', 'amount']].query('customer_id == "CS018205000001" & (amount >= 1000 | quantity >=5)')
is used to select a subset of rows from a Pandas DataFrame calleddf_receipt
, where thecustomer_id
column is equal to a specific value (CS018205000001
) and either theamount
column is greater than or equal to 1000 or thequantity
column is greater than or equal to 5.
The codedf_receipt[['sales_ymd', 'customer_id', 'product_cd', 'quantity', 'amount']]
is used to select a subset of columns from the DataFramedf_receipt
. Specifically, it selects the columns namedsales_ymd
,customer_id
,product_cd
,quantity
, andamount
.
The.query()
method is then used to filter the rows of the DataFrame based on multiple conditions. The&
symbol is used to indicate a logical AND operation between the two conditions. The first condition is that thecustomer_id
column must be equal to the value"CS018205000001"
. The second condition is enclosed in parentheses and contains two sub-conditions separated by the|
symbol, which indicates a logical OR operation. The first sub-condition is that theamount
column must be greater than or equal to 1000. The second sub-condition is that thequantity
column must be greater than or equal to 5.
The resulting DataFrame contains only the rows where both thecustomer_id
is equal to the specified value and either theamount
is greater than or equal to 1000 or thequantity
is greater than or equal to 5.
P-007: From the receipt details data (df_receipt), specify the columns in order of sales date (sales_ymd), customer ID (customer_id), product code (product_cd) and sales amount (amount) and extract data that satisfy all the following conditions.
- Customer ID (customer_id) is โCS018205000001โ.
- Sales amount (amount) is between 1,000 and 2,000.
df_receipt[['sales_ymd', 'customer_id', 'product_cd', 'amount']] \
.query('customer_id == "CS018205000001" & 1000 <= amount <= 2000')
sales_ymd | customer_id | product_cd | amount | |
---|---|---|---|---|
72254 | 20180911 | CS018205000001 | P071401005 | 1100 |
Commentary :
The codedf_receipt[['sales_ymd', 'customer_id', 'product_cd', 'amount']] \ .query('customer_id == "CS018205000001" & 1000 <= amount <= 2000')
is used to select a subset of rows from a Pandas DataFrame calleddf_receipt
, where thecustomer_id
column is equal to a specific value (CS018205000001
) and theamount
column is between 1000 and 2000 (inclusive).
The codedf_receipt[['sales_ymd', 'customer_id', 'product_cd', 'amount']]
is used to select a subset of columns from the DataFramedf_receipt
. Specifically, it selects the columns namedsales_ymd
,customer_id
,product_cd
, andamount
.
The.query()
method is then used to filter the rows of the DataFrame based on multiple conditions. The&
symbol is used to indicate a logical AND operation between the two conditions. The first condition is that thecustomer_id
column must be equal to the value"CS018205000001"
. The second condition specifies a range of values for theamount
column, using the<=
and>=
comparison operators.
The resulting DataFrame contains only the rows where both thecustomer_id
is equal to the specified value and theamount
is between 1000 and 2000 (inclusive).
P-008: From the receipt details data (df_receipt), specify the columns in order of sales date (sales_ymd), customer ID (customer_id), product code (product_cd) and sales amount (amount), and extract data satisfying all the following conditions.
- Customer ID (customer_id) is โCS018205000001โ.
- Product code (product_cd) is other than โP071401019โ.
df_receipt[['sales_ymd', 'customer_id', 'product_cd', 'amount']] \
.query('customer_id == "CS018205000001" & product_cd != "P071401019"')
sales_ymd | customer_id | product_cd | amount | |
---|---|---|---|---|
36 | 20180911 | CS018205000001 | P071401012 | 2200 |
9843 | 20180414 | CS018205000001 | P060104007 | 600 |
21110 | 20170614 | CS018205000001 | P050206001 | 990 |
27673 | 20170614 | CS018205000001 | P060702015 | 108 |
27840 | 20190216 | CS018205000001 | P071005024 | 102 |
28757 | 20180414 | CS018205000001 | P071101002 | 278 |
39256 | 20190226 | CS018205000001 | P070902035 | 168 |
58121 | 20190924 | CS018205000001 | P060805001 | 495 |
68117 | 20190226 | CS018205000001 | P071401020 | 2200 |
72254 | 20180911 | CS018205000001 | P071401005 | 1100 |
88508 | 20190216 | CS018205000001 | P040101002 | 218 |
91525 | 20190924 | CS018205000001 | P091503001 | 280 |
Commentary :
The codedf_receipt[['sales_ymd', 'customer_id', 'product_cd', 'amount']] \ .query('customer_id == "CS018205000001" & product_cd != "P071401019"')
is used to select a subset of rows from a Pandas DataFrame calleddf_receipt
, where thecustomer_id
column is equal to a specific value (CS018205000001
) and theproduct_cd
column is not equal to a specific value (P071401019
).
The codedf_receipt[['sales_ymd', 'customer_id', 'product_cd', 'amount']]
is used to select a subset of columns from the DataFramedf_receipt
. Specifically, it selects the columns namedsales_ymd
,customer_id
,product_cd
, andamount
.
The.query()
method is then used to filter the rows of the DataFrame based on multiple conditions. The&
symbol is used to indicate a logical AND operation between the two conditions. The first condition is that thecustomer_id
column must be equal to the value"CS018205000001"
. The second condition is that theproduct_cd
column must not be equal to the value"P071401019"
, which is achieved using the!=
comparison operator.
The resulting DataFrame contains only the rows where both thecustomer_id
is equal to the specified value and theproduct_cd
is not equal to the specified value.
P-009: In the following process, rewrite OR to AND without changing the output result.
df_store.query('not(prefecture_cd == "13" | floor_area > 900)')
df_store.query('prefecture_cd != "13" & floor_area <= 900')
store_cd | store_name | prefecture_cd | prefecture | address | address_kana | tel_no | longitude | latitude | floor_area | |
---|---|---|---|---|---|---|---|---|---|---|
18 | S14046 | ๅๅฑฑ็ฐๅบ | 14 | ็ฅๅฅๅท็ | ็ฅๅฅๅท็ๆจชๆตๅธ้ฝ็ญๅบๅๅฑฑ็ฐไธไธ็ฎ | ใซใใฌใฏใฑใณใจใณใใใทใใ ใญใฏใญใฟใคใใฟใคใใใงใฆใก | 045-123-4049 | 139.5916 | 35.56189 | 831.0 |
20 | S14011 | ๆฅๅๆฌ็บๅบ | 14 | ็ฅๅฅๅท็ | ็ฅๅฅๅท็ๆจชๆตๅธๆธฏๅๅบๆฅๅๆฌ็บๅไธ็ฎ | ใซใใฌใฏใฑใณใจใณใใใทใณใฆใใฏใฏใใจใทใใณใใงใฆใจใณใใงใฆใก | 045-123-4033 | 139.6316 | 35.54655 | 890.0 |
38 | S12013 | ็ฟๅฟ้ๅบ | 12 | ๅ่็ | ๅ่็็ฟๅฟ้ๅธ่ๅไธไธ็ฎ | ใใใฑใณใใฉใทใใทใทใใพใใคใใใงใฆใก | 047-123-4002 | 140.0220 | 35.66122 | 808.0 |
Commentary :
The codedf_store.query('prefecture_cd != "13" & floor_area <= 900')
is used to filter rows from a Pandas DataFrame calleddf_store
, where theprefecture_cd
column is not equal to a specific value (13
) AND thefloor_area
column is less than or equal to a specific value (900
).
The.query()
method is used to filter the rows of the DataFrame based on a Boolean expression. In this case, the Boolean expression is'prefecture_cd != "13" & floor_area <= 900'
.
The!=
operator is used to check if each value in theprefecture_cd
column is not equal to the value"13"
. The&
operator is used to indicate a logical AND operation between this condition and the second condition, which checks if the values in thefloor_area
column are less than or equal to900
.
The resulting DataFrame contains only the rows where both conditions are True.
P-010: From the shop data (df_store), extract all items whose shop code (store_cd) starts with โS14โ and display 10 items.
df_store.query("store_cd.str.startswith('S14')", engine='python').head(10)
store_cd | store_name | prefecture_cd | prefecture | address | address_kana | tel_no | longitude | latitude | floor_area | |
---|---|---|---|---|---|---|---|---|---|---|
2 | S14010 | ่ๅๅบ | 14 | ็ฅๅฅๅท็ | ็ฅๅฅๅท็ๆจชๆตๅธๆธฏๅๅบ่ๅไธไธ็ฎ | ใซใใฌใฏใฑใณใจใณใใใทใณใฆใใฏใฏใญใฏใใคใใใงใฆใก | 045-123-4032 | 139.6326 | 35.50049 | 1732.0 |
3 | S14033 | ้ฟไน ๅๅบ | 14 | ็ฅๅฅๅท็ | ็ฅๅฅๅท็ๆจชๆตๅธ็ฌ่ฐทๅบ้ฟไน ๅ่ฅฟไธไธ็ฎ | ใซใใฌใฏใฑใณใจใณใใใทใปใคใฏใขใฏใฏใใทใคใใใงใฆใก | 045-123-4043 | 139.4961 | 35.45918 | 1495.0 |
4 | S14036 | ็ธๆจกๅไธญๅคฎๅบ | 14 | ็ฅๅฅๅท็ | ็ฅๅฅๅท็็ธๆจกๅๅธไธญๅคฎไบไธ็ฎ | ใซใใฌใฏใฑใณใตใฌใใใฉใทใใฅใฆใชใฆใใใงใฆใก | 042-123-4045 | 139.3716 | 35.57327 | 1679.0 |
7 | S14040 | ้ทๆดฅ็ฐๅบ | 14 | ็ฅๅฅๅท็ | ็ฅๅฅๅท็ๆจชๆตๅธ็ทๅบ้ทๆดฅ็ฐใฟใชใฟๅฐไบไธ็ฎ | ใซใใฌใฏใฑใณใจใณใใใทใใใชใฏใใฌใใฟใใใใใคใดใใงใฆใก | 045-123-4046 | 139.4994 | 35.52398 | 1548.0 |
9 | S14050 | ้ฟไน ๅ่ฅฟๅบ | 14 | ็ฅๅฅๅท็ | ็ฅๅฅๅท็ๆจชๆตๅธ็ฌ่ฐทๅบ้ฟไน ๅ่ฅฟไธไธ็ฎ | ใซใใฌใฏใฑใณใจใณใใใทใปใคใฏใขใฏใฏใใทใคใใใงใฆใก | 045-123-4053 | 139.4961 | 35.45918 | 1830.0 |
12 | S14028 | ไบใๆฉๅบ | 14 | ็ฅๅฅๅท็ | ็ฅๅฅๅท็ๆจชๆตๅธ็ฌ่ฐทๅบไบใๆฉ็บ | ใซใใฌใฏใฑใณใจใณใใใทใปใคใฏใใฟใใใทใใงใฆ | 045-123-4042 | 139.4963 | 35.46304 | 1574.0 |
16 | S14012 | ๆฌ็งๅ็ฐๅบ | 14 | ็ฅๅฅๅท็ | ็ฅๅฅๅท็ๆจชๆตๅธไธญๅบๆฌ็งๅ็ฐ | ใซใใฌใฏใฑใณใจใณใใใทใใซใฏใใณใขใฏใฏใ | 045-123-4034 | 139.6582 | 35.42156 | 1341.0 |
18 | S14046 | ๅๅฑฑ็ฐๅบ | 14 | ็ฅๅฅๅท็ | ็ฅๅฅๅท็ๆจชๆตๅธ้ฝ็ญๅบๅๅฑฑ็ฐไธไธ็ฎ | ใซใใฌใฏใฑใณใจใณใใใทใใ ใญใฏใญใฟใคใใฟใคใใใงใฆใก | 045-123-4049 | 139.5916 | 35.56189 | 831.0 |
19 | S14022 | ้ๅญๅบ | 14 | ็ฅๅฅๅท็ | ็ฅๅฅๅท็้ๅญๅธ้ๅญไธไธ็ฎ | ใซใใฌใฏใฑใณใบใทใทใบใทใคใใใงใฆใก | 046-123-4036 | 139.5789 | 35.29642 | 1838.0 |
20 | S14011 | ๆฅๅๆฌ็บๅบ | 14 | ็ฅๅฅๅท็ | ็ฅๅฅๅท็ๆจชๆตๅธๆธฏๅๅบๆฅๅๆฌ็บๅไธ็ฎ | ใซใใฌใฏใฑใณใจใณใใใทใณใฆใใฏใฏใใจใทใใณใใงใฆใจใณใใงใฆใก | 045-123-4033 | 139.6316 | 35.54655 | 890.0 |
Commentary :
The codedf_store.query("store_cd.str.startswith('S14')", engine='python').head(10)
is used to filter rows from a Pandas DataFrame calleddf_store
, where thestore_cd
column starts with a specific string (S14
).
The.query()
method is used to filter the rows of the DataFrame based on a Boolean expression. In this case, the Boolean expression is"store_cd.str.startswith('S14')"
. This expression is written in Python syntax because theengine
parameter is set to'python'
. The expression checks whether thestore_cd
column starts with the string'S14'
.
Thestr.startswith()
method is a string method in Python that returnsTrue
if the string starts with the specified prefix. In this case, thestr.startswith('S14')
method is applied to each element in thestore_cd
column.
The resulting DataFrame contains only the rows where thestore_cd
column starts with the string'S14'
.
The.head(10)
method is used to return only the first 10 rows of the resulting DataFrame.
P-011: From the customer data (df_customer), extract all items whose customer ID (customer_id) ends with 1, and display 10 items.
df_customer.query("customer_id.str.endswith('1')", engine='python').head(10)
customer_id | customer_name | gender_cd | gender | birth_day | age | postal_cd | address | application_store_cd | application_date | status_cd | |
---|---|---|---|---|---|---|---|---|---|---|---|
1 | CS037613000071 | ๅ ญ่ง ้ ๅฝฆ | 9 | ไธๆ | 1952-04-01 | 66 | 136-0076 | ๆฑไบฌ้ฝๆฑๆฑๅบๅ็ ********** | S13037 | 20150414 | 0-00000000-0 |
3 | CS028811000001 | ๅ ไบ ใใใ | 1 | ๅฅณๆง | 1933-03-27 | 86 | 245-0016 | ็ฅๅฅๅท็ๆจชๆตๅธๆณๅบๅๆณ็บ********** | S14028 | 20160115 | 0-00000000-0 |
14 | CS040412000191 | ๅทไบ ้ๆต | 1 | ๅฅณๆง | 1977-01-05 | 42 | 226-0021 | ็ฅๅฅๅท็ๆจชๆตๅธ็ทๅบๅๅ ซๆ็บ********** | S14040 | 20151101 | 1-20091025-4 |
31 | CS028314000011 | ๅฐ่ ใใใ | 1 | ๅฅณๆง | 1983-11-26 | 35 | 246-0038 | ็ฅๅฅๅท็ๆจชๆตๅธ็ฌ่ฐทๅบๅฎฎๆฒข********** | S14028 | 20151123 | 1-20080426-5 |
56 | CS039212000051 | ่คๅณถ ๆตๆขจ้ฆ | 1 | ๅฅณๆง | 1997-02-03 | 22 | 166-0001 | ๆฑไบฌ้ฝๆไธฆๅบ้ฟไฝ่ฐทๅ********** | S13039 | 20171121 | 1-20100215-4 |
59 | CS015412000111 | ๆพๅฑ ๅฅๆ | 1 | ๅฅณๆง | 1972-10-04 | 46 | 136-0071 | ๆฑไบฌ้ฝๆฑๆฑๅบไบๆธ********** | S13015 | 20150629 | 0-00000000-0 |
63 | CS004702000041 | ้ๅณถ ๆด | 0 | ็ทๆง | 1943-08-24 | 75 | 176-0022 | ๆฑไบฌ้ฝ็ทด้ฆฌๅบๅๅฑฑ********** | S13004 | 20170218 | 0-00000000-0 |
74 | CS041515000001 | ๆ ็ฐ ๅๅค | 1 | ๅฅณๆง | 1967-01-02 | 52 | 206-0001 | ๆฑไบฌ้ฝๅคๆฉๅธๅ็ฐ********** | S13041 | 20160422 | E-20100803-F |
85 | CS029313000221 | ๅๆก ใฒใใ | 1 | ๅฅณๆง | 1987-06-19 | 31 | 279-0011 | ๅ่็ๆตฆๅฎๅธ็พๆต********** | S12029 | 20180810 | 0-00000000-0 |
102 | CS034312000071 | ๆๆ ๅฅๅคฎ | 1 | ๅฅณๆง | 1980-09-20 | 38 | 213-0026 | ็ฅๅฅๅท็ๅทๅดๅธ้ซๆดฅๅบไน ๆซ********** | S14034 | 20160106 | 0-00000000-0 |
Commentary :
This code is selecting a subset of data from a Pandas DataFrame nameddf_customer
. The subset is filtered based on a condition specified in thequery()
method.
The condition is defined by the string"customer_id.str.endswith('1')"
, which is passed as an argument to thequery()
method. This condition filters the rows of thedf_customer
DataFrame to only include those where thecustomer_id
column ends with the character'1'
.
Theengine='python'
parameter is optional and specifies that the Python engine should be used to evaluate the condition. This is necessary when the condition contains string operations, as the default engine forquery()
method (pandas.eval) may not support all string operations.
Finally, thehead(10)
method is used to return the first 10 rows of the filtered DataFrame. This is optional and is used here to limit the output to a manageable size.
P-012: From shop data (df_store), display all items with โๆจชๆตๅธ(Yokohama-city)โ in the address.
df_store.query("address.str.contains('ๆจชๆตๅธ')", engine='python')
store_cd | store_name | prefecture_cd | prefecture | address | address_kana | tel_no | longitude | latitude | floor_area | |
---|---|---|---|---|---|---|---|---|---|---|
2 | S14010 | ่ๅๅบ | 14 | ็ฅๅฅๅท็ | ็ฅๅฅๅท็ๆจชๆตๅธๆธฏๅๅบ่ๅไธไธ็ฎ | ใซใใฌใฏใฑใณใจใณใใใทใณใฆใใฏใฏใญใฏใใคใใใงใฆใก | 045-123-4032 | 139.6326 | 35.50049 | 1732.0 |
3 | S14033 | ้ฟไน ๅๅบ | 14 | ็ฅๅฅๅท็ | ็ฅๅฅๅท็ๆจชๆตๅธ็ฌ่ฐทๅบ้ฟไน ๅ่ฅฟไธไธ็ฎ | ใซใใฌใฏใฑใณใจใณใใใทใปใคใฏใขใฏใฏใใทใคใใใงใฆใก | 045-123-4043 | 139.4961 | 35.45918 | 1495.0 |
7 | S14040 | ้ทๆดฅ็ฐๅบ | 14 | ็ฅๅฅๅท็ | ็ฅๅฅๅท็ๆจชๆตๅธ็ทๅบ้ทๆดฅ็ฐใฟใชใฟๅฐไบไธ็ฎ | ใซใใฌใฏใฑใณใจใณใใใทใใใชใฏใใฌใใฟใใใใใคใดใใงใฆใก | 045-123-4046 | 139.4994 | 35.52398 | 1548.0 |
9 | S14050 | ้ฟไน ๅ่ฅฟๅบ | 14 | ็ฅๅฅๅท็ | ็ฅๅฅๅท็ๆจชๆตๅธ็ฌ่ฐทๅบ้ฟไน ๅ่ฅฟไธไธ็ฎ | ใซใใฌใฏใฑใณใจใณใใใทใปใคใฏใขใฏใฏใใทใคใใใงใฆใก | 045-123-4053 | 139.4961 | 35.45918 | 1830.0 |
12 | S14028 | ไบใๆฉๅบ | 14 | ็ฅๅฅๅท็ | ็ฅๅฅๅท็ๆจชๆตๅธ็ฌ่ฐทๅบไบใๆฉ็บ | ใซใใฌใฏใฑใณใจใณใใใทใปใคใฏใใฟใใใทใใงใฆ | 045-123-4042 | 139.4963 | 35.46304 | 1574.0 |
16 | S14012 | ๆฌ็งๅ็ฐๅบ | 14 | ็ฅๅฅๅท็ | ็ฅๅฅๅท็ๆจชๆตๅธไธญๅบๆฌ็งๅ็ฐ | ใซใใฌใฏใฑใณใจใณใใใทใใซใฏใใณใขใฏใฏใ | 045-123-4034 | 139.6582 | 35.42156 | 1341.0 |
18 | S14046 | ๅๅฑฑ็ฐๅบ | 14 | ็ฅๅฅๅท็ | ็ฅๅฅๅท็ๆจชๆตๅธ้ฝ็ญๅบๅๅฑฑ็ฐไธไธ็ฎ | ใซใใฌใฏใฑใณใจใณใใใทใใ ใญใฏใญใฟใคใใฟใคใใใงใฆใก | 045-123-4049 | 139.5916 | 35.56189 | 831.0 |
20 | S14011 | ๆฅๅๆฌ็บๅบ | 14 | ็ฅๅฅๅท็ | ็ฅๅฅๅท็ๆจชๆตๅธๆธฏๅๅบๆฅๅๆฌ็บๅไธ็ฎ | ใซใใฌใฏใฑใณใจใณใใใทใณใฆใใฏใฏใใจใทใใณใใงใฆใจใณใใงใฆใก | 045-123-4033 | 139.6316 | 35.54655 | 890.0 |
26 | S14048 | ไธญๅทไธญๅคฎๅบ | 14 | ็ฅๅฅๅท็ | ็ฅๅฅๅท็ๆจชๆตๅธ้ฝ็ญๅบไธญๅทไธญๅคฎไบไธ็ฎ | ใซใใฌใฏใฑใณใจใณใใใทใใ ใญใฏใใซใฌใฏใใฅใฆใชใฆใใใงใฆใก | 045-123-4051 | 139.5758 | 35.54912 | 1657.0 |
40 | S14042 | ๆฐๅฑฑไธๅบ | 14 | ็ฅๅฅๅท็ | ็ฅๅฅๅท็ๆจชๆตๅธไธญๅบๆฐๅฑฑไธไบไธ็ฎ | ใซใใฌใฏใฑใณใจใณใใใทใใซใฏใทใณใคใใทใฟใใใงใฆใก | 045-123-4047 | 139.6593 | 35.43894 | 1044.0 |
52 | S14006 | ่ใ่ฐทๅบ | 14 | ็ฅๅฅๅท็ | ็ฅๅฅๅท็ๆจชๆตๅธ้ฝ็ญๅบ่ใ่ฐท | ใซใใฌใฏใฑใณใจใณใใใทใใ ใญใฏใฏใบใฌใค | 045-123-4031 | 139.5633 | 35.53573 | 1886.0 |
Commentary :
Specifically, the condition is defined by the string"address.str.contains('ๆจชๆตๅธ')"
, which is passed as an argument toquery()
. This string is a boolean expression that checks if theaddress
column ofdf_store
contains the substring'ๆจชๆตๅธ'
.
Thestr.contains()
method is a Pandas string method that returns a boolean array indicating whether each element of a given string column contains a specified substring. In this case, it is applied to theaddress
column ofdf_store
.
Theengine='python'
argument specifies that the filtering operation should be performed using Python's built-ineval()
function, rather than the default numexpr engine. This is necessary when using certain types of string operations in the query expression, such asstr.contains()
, which are not supported by the numexpr engine.
P-013: From the customer data (df_customer), extract all data whose status code (status_cd) begins with the letters A to F and display 10 items.
df_customer.query("status_cd.str.contains(r'^[A-F]')",
engine='python').head(10)
customer_id | customer_name | gender_cd | gender | birth_day | age | postal_cd | address | application_store_cd | application_date | status_cd | |
---|---|---|---|---|---|---|---|---|---|---|---|
2 | CS031415000172 | ๅฎๅค็ฐ ่ฒด็พๅญ | 1 | ๅฅณๆง | 1976-10-04 | 42 | 151-0053 | ๆฑไบฌ้ฝๆธ่ฐทๅบไปฃใ ๆจ********** | S13031 | 20150529 | D-20100325-C |
6 | CS015414000103 | ๅฅฅ้ ้ฝๅญ | 1 | ๅฅณๆง | 1977-08-09 | 41 | 136-0073 | ๆฑไบฌ้ฝๆฑๆฑๅบๅ็ ********** | S13015 | 20150722 | B-20100609-B |
12 | CS011215000048 | ่ฆ็ฐ ๆฒ่ถ | 1 | ๅฅณๆง | 1992-02-01 | 27 | 223-0062 | ็ฅๅฅๅท็ๆจชๆตๅธๆธฏๅๅบๆฅๅๆฌ็บ********** | S14011 | 20150228 | C-20100421-9 |
15 | CS029415000023 | ๆข ็ฐ ้็ฉ | 1 | ๅฅณๆง | 1976-01-17 | 43 | 279-0043 | ๅ่็ๆตฆๅฎๅธๅฏๅฃซ่ฆ********** | S12029 | 20150610 | D-20100918-E |
21 | CS035415000029 | ๅฏบๆฒข ็ๅธ | 9 | ไธๆ | 1977-09-27 | 41 | 158-0096 | ๆฑไบฌ้ฝไธ็ฐ่ฐทๅบ็ๅทๅฐ********** | S13035 | 20141220 | F-20101029-F |
32 | CS031415000106 | ๅฎ้ ็ฑ็พๅญ | 1 | ๅฅณๆง | 1970-02-26 | 49 | 151-0053 | ๆฑไบฌ้ฝๆธ่ฐทๅบไปฃใ ๆจ********** | S13031 | 20150201 | F-20100511-E |
33 | CS029215000025 | ็ณๅ ็พๅธ | 1 | ๅฅณๆง | 1993-09-28 | 25 | 279-0022 | ๅ่็ๆตฆๅฎๅธไปๅท********** | S12029 | 20150708 | B-20100820-C |
40 | CS033605000005 | ็ช่ก ้ๅคช | 0 | ็ทๆง | 1955-12-05 | 63 | 246-0031 | ็ฅๅฅๅท็ๆจชๆตๅธ็ฌ่ฐทๅบ็ฌ่ฐท********** | S14033 | 20150425 | F-20100917-E |
44 | CS033415000229 | ๆฟๅฃ ่ใ ็พ | 1 | ๅฅณๆง | 1977-11-07 | 41 | 246-0021 | ็ฅๅฅๅท็ๆจชๆตๅธ็ฌ่ฐทๅบไบใๆฉ็บ********** | S14033 | 20150712 | F-20100326-E |
53 | CS008415000145 | ้ป่ฐท ้บป็ท | 1 | ๅฅณๆง | 1977-06-27 | 41 | 157-0067 | ๆฑไบฌ้ฝไธ็ฐ่ฐทๅบๅๅค่ฆ********** | S13008 | 20150829 | F-20100622-F |
Commentary :df_customer
is a DataFrame object..query()
is a method provided by Pandas for filtering rows based on a specific condition. The condition is specified as a string argument.
The condition in this case is"status_cd.str.contains(r'^[A-F]')"
. This uses thestr.contains()
method to check whether thestatus_cd
column of the DataFrame contains any strings that start with the letters A through F (inclusive). Ther
before the regular expression pattern'^[A-F]'
indicates a raw string literal, which ensures that the backslash character (\
) is interpreted literally and not as an escape character.
Theengine='python'
argument specifies that the filtering should be done using Python's built-in regular expression engine, rather than the default engine used by Pandas. This can be useful in cases where the default engine doesn't support certain regex features or is too slow.
The.head(10)
method is used to return only the first 10 rows of the filtered DataFrame.
So, overall, this code is filtering thedf_customer
DataFrame to only include rows where thestatus_cd
column starts with a letter from A to F, and then returning the first 10 rows of the resulting DataFrame.
P-014: From the customer data (df_customer), extract all data items whose status code (status_cd) ends with the numbers 1 to 9, and display 10 items.
# A regex option can also be added (if False, it is treated as a string as is, not as a regular expression).
df_customer.query("status_cd.str.contains(r'[1-9]$', regex=True)",
engine='python').head(10)
customer_id | customer_name | gender_cd | gender | birth_day | age | postal_cd | address | application_store_cd | application_date | status_cd | |
---|---|---|---|---|---|---|---|---|---|---|---|
4 | CS001215000145 | ็ฐๅด ็พ็ด | 1 | ๅฅณๆง | 1995-03-29 | 24 | 144-0055 | ๆฑไบฌ้ฝๅคง็ฐๅบไปฒๅ ญ้ท********** | S13001 | 20170605 | 6-20090929-2 |
9 | CS033513000180 | ๅฎๆ ้ฅ | 1 | ๅฅณๆง | 1962-07-11 | 56 | 241-0823 | ็ฅๅฅๅท็ๆจชๆตๅธๆญๅบๅ้จ็บ********** | S14033 | 20150728 | 6-20080506-5 |
12 | CS011215000048 | ่ฆ็ฐ ๆฒ่ถ | 1 | ๅฅณๆง | 1992-02-01 | 27 | 223-0062 | ็ฅๅฅๅท็ๆจชๆตๅธๆธฏๅๅบๆฅๅๆฌ็บ********** | S14011 | 20150228 | C-20100421-9 |
14 | CS040412000191 | ๅทไบ ้ๆต | 1 | ๅฅณๆง | 1977-01-05 | 42 | 226-0021 | ็ฅๅฅๅท็ๆจชๆตๅธ็ทๅบๅๅ ซๆ็บ********** | S14040 | 20151101 | 1-20091025-4 |
16 | CS009315000023 | ็ๅท ๆไธ | 1 | ๅฅณๆง | 1980-04-15 | 38 | 154-0012 | ๆฑไบฌ้ฝไธ็ฐ่ฐทๅบ้งๆฒข********** | S13009 | 20150319 | 5-20080322-1 |
22 | CS015315000033 | ็ฆๅฃซ ็ๅฅๅญ | 1 | ๅฅณๆง | 1983-03-17 | 36 | 135-0043 | ๆฑไบฌ้ฝๆฑๆฑๅบๅกฉๆต********** | S13015 | 20141024 | 4-20080219-3 |
23 | CS023513000066 | ็ฅๆธ ใใ | 1 | ๅฅณๆง | 1961-12-17 | 57 | 210-0005 | ็ฅๅฅๅท็ๅทๅดๅธๅทๅดๅบๆฑ็ฐ็บ********** | S14023 | 20150915 | 5-20100524-9 |
24 | CS035513000134 | ๅธๅท ็พๅธ | 1 | ๅฅณๆง | 1960-03-27 | 59 | 156-0053 | ๆฑไบฌ้ฝไธ็ฐ่ฐทๅบๆก********** | S13035 | 20150227 | 8-20100711-9 |
27 | CS001515000263 | ้ซๆพ ๅค็ฉบ | 1 | ๅฅณๆง | 1962-11-09 | 56 | 144-0051 | ๆฑไบฌ้ฝๅคง็ฐๅบ่ฅฟ่ฒ็ฐ********** | S13001 | 20160812 | 1-20100804-1 |
28 | CS040314000027 | ้ถด็ฐ ใใฟใพใ | 9 | ไธๆ | 1986-03-26 | 33 | 226-0027 | ็ฅๅฅๅท็ๆจชๆตๅธ็ทๅบ้ทๆดฅ็ฐ********** | S14040 | 20150122 | 2-20080426-4 |
Commentary :df_customer
is a DataFrame object..query()
is a method provided by Pandas for filtering rows based on a specific condition. The condition is specified as a string argument.
The condition in this case is"status_cd.str.contains(r'[1-9]$', regex=True)"
. This uses thestr.contains()
method to check whether thestatus_cd
column of the DataFrame contains any strings that end with a digit from 1 to 9 (inclusive). The$
character represents the end of the string, and theregex=True
argument indicates that the pattern should be interpreted as a regular expression.
Theengine='python'
argument specifies that the filtering should be done using Python's built-in regular expression engine, rather than the default engine used by Pandas. This can be useful in cases where the default engine doesn't support certain regex features or is too slow.
The.head(10)
method is used to return only the first 10 rows of the filtered DataFrame.So, overall, this code is filtering thedf_customer
DataFrame to only include rows where thestatus_cd
column ends with a digit from 1 to 9, and then returning the first 10 rows of the resulting DataFrame.
P-015: From the customer data (df_customer), extract all data items whose status code (status_cd) begins with the letters A to F and ends with the numbers 1 to 9, and display 10 items.
df_customer.query("status_cd.str.contains(r'^[A-F].*[1-9]$')",
engine='python').head(10)
customer_id | customer_name | gender_cd | gender | birth_day | age | postal_cd | address | application_store_cd | application_date | status_cd | |
---|---|---|---|---|---|---|---|---|---|---|---|
12 | CS011215000048 | ่ฆ็ฐ ๆฒ่ถ | 1 | ๅฅณๆง | 1992-02-01 | 27 | 223-0062 | ็ฅๅฅๅท็ๆจชๆตๅธๆธฏๅๅบๆฅๅๆฌ็บ********** | S14011 | 20150228 | C-20100421-9 |
68 | CS022513000105 | ๅณถๆ ่ฒด็พๅญ | 1 | ๅฅณๆง | 1962-03-12 | 57 | 249-0002 | ็ฅๅฅๅท็้ๅญๅธๅฑฑใฎๆ น********** | S14022 | 20150320 | A-20091115-7 |
71 | CS001515000096 | ๆฐด้ ้ฝๅญ | 9 | ไธๆ | 1960-11-29 | 58 | 144-0053 | ๆฑไบฌ้ฝๅคง็ฐๅบ่ฒ็ฐๆฌ็บ********** | S13001 | 20150614 | A-20100724-7 |
122 | CS013615000053 | ่ฅฟ่ ๅญฃ่กฃ | 1 | ๅฅณๆง | 1953-10-18 | 65 | 261-0026 | ๅ่็ๅ่ๅธ็พๆตๅบๅนๅผต่ฅฟ********** | S12013 | 20150128 | B-20100329-6 |
144 | CS020412000161 | ๅฐๅฎฎ ่ซ | 1 | ๅฅณๆง | 1974-05-21 | 44 | 174-0042 | ๆฑไบฌ้ฝๆฟๆฉๅบๆฑๅไธ********** | S13020 | 20150822 | B-20081021-3 |
178 | CS001215000097 | ็ซนไธญ ใใใฟ | 1 | ๅฅณๆง | 1990-07-25 | 28 | 146-0095 | ๆฑไบฌ้ฝๅคง็ฐๅบๅคๆฉๅท********** | S13001 | 20170315 | A-20100211-2 |
252 | CS035212000007 | ๅ ๆ ๆตๆขจ้ฆ | 1 | ๅฅณๆง | 1990-12-04 | 28 | 152-0023 | ๆฑไบฌ้ฝ็ฎ้ปๅบๅ ซ้ฒ********** | S13035 | 20151013 | B-20101018-6 |
259 | CS002515000386 | ้็ฐ ใณใฆ | 1 | ๅฅณๆง | 1963-05-30 | 55 | 185-0013 | ๆฑไบฌ้ฝๅฝๅๅฏบๅธ่ฅฟๆใฑ็ชช********** | S13002 | 20160410 | C-20100127-8 |
293 | CS001615000372 | ็จฒๅฃ ๅฏฟใ ่ฑ | 1 | ๅฅณๆง | 1956-10-29 | 62 | 144-0035 | ๆฑไบฌ้ฝๅคง็ฐๅบๅ่ฒ็ฐ********** | S13001 | 20170403 | A-20100104-1 |
297 | CS032512000121 | ๆพไบ ็ฅไธ | 1 | ๅฅณๆง | 1962-09-04 | 56 | 210-0011 | ็ฅๅฅๅท็ๅทๅดๅธๅทๅดๅบๅฏๅฃซ่ฆ********** | S13032 | 20150727 | A-20100103-5 |
Commentary :
This code uses the Pandas library in Python to filter rows from a DataFrame nameddf_customer
. The filter is based on a condition specified in thequery()
method, which takes a string argument representing a Boolean expression.status_cd
: This is assumed to be a column in thedf_customer
DataFrame..
: This is the dot notation used to access a method or attribute of an object in Python.str
: This refers to the string methods of thestatus_cd
column.contains()
: This is a string method that checks whether a given substring or regular expression is present in each element of the column.r'^[A-F].*[1-9]$'
: This is a regular expression pattern that matches strings that start with a letter A through F (inclusive) and end with a digit from 1 through 9 (inclusive). Ther
prefix before the pattern indicates that it is a raw string, which means that backslashes are treated as literal backslashes rather than escape characters.
Thequery()
method is also specifying anengine
argument with the value'python'
. This is because the default engine used by Pandas isnumexpr
, which can be faster for some types of queries but may not support all types of regular expressions. Using the'python'
engine ensures that the regular expression is handled by the Python regex module.
Finally, the.head(10)
method call limits the output to the first 10 rows that match the filter condition.
P-016: From the shop data (df_store), display all items of data where the telephone number (tel_no) is 3 digits โ 3 digits โ 4 digits.
df_store.query("tel_no.str.contains(r'^[0-9]{3}-[0-9]{3}-[0-9]{4}$')",
engine='python')
store_cd | store_name | prefecture_cd | prefecture | address | address_kana | tel_no | longitude | latitude | floor_area | |
---|---|---|---|---|---|---|---|---|---|---|
0 | S12014 | ๅ่ๅฐๅบ | 12 | ๅ่็ | ๅ่็ๅ่ๅธ็จฒๆฏๅบๅ่ๅฐไธไธ็ฎ | ใใใฑใณใใใทใคใใฒใฏใใฐใตใใคใคใใใงใฆใก | 043-123-4003 | 140.1180 | 35.63559 | 1698.0 |
1 | S13002 | ๅฝๅๅฏบๅบ | 13 | ๆฑไบฌ้ฝ | ๆฑไบฌ้ฝๅฝๅๅฏบๅธๆฌๅคไบไธ็ฎ | ใใฆใญใงใฆใใณใฏใใณใธใทใใณใใใใงใฆใก | 042-123-4008 | 139.4802 | 35.70566 | 1735.0 |
2 | S14010 | ่ๅๅบ | 14 | ็ฅๅฅๅท็ | ็ฅๅฅๅท็ๆจชๆตๅธๆธฏๅๅบ่ๅไธไธ็ฎ | ใซใใฌใฏใฑใณใจใณใใใทใณใฆใใฏใฏใญใฏใใคใใใงใฆใก | 045-123-4032 | 139.6326 | 35.50049 | 1732.0 |
3 | S14033 | ้ฟไน ๅๅบ | 14 | ็ฅๅฅๅท็ | ็ฅๅฅๅท็ๆจชๆตๅธ็ฌ่ฐทๅบ้ฟไน ๅ่ฅฟไธไธ็ฎ | ใซใใฌใฏใฑใณใจใณใใใทใปใคใฏใขใฏใฏใใทใคใใใงใฆใก | 045-123-4043 | 139.4961 | 35.45918 | 1495.0 |
4 | S14036 | ็ธๆจกๅไธญๅคฎๅบ | 14 | ็ฅๅฅๅท็ | ็ฅๅฅๅท็็ธๆจกๅๅธไธญๅคฎไบไธ็ฎ | ใซใใฌใฏใฑใณใตใฌใใใฉใทใใฅใฆใชใฆใใใงใฆใก | 042-123-4045 | 139.3716 | 35.57327 | 1679.0 |
7 | S14040 | ้ทๆดฅ็ฐๅบ | 14 | ็ฅๅฅๅท็ | ็ฅๅฅๅท็ๆจชๆตๅธ็ทๅบ้ทๆดฅ็ฐใฟใชใฟๅฐไบไธ็ฎ | ใซใใฌใฏใฑใณใจใณใใใทใใใชใฏใใฌใใฟใใใใใคใดใใงใฆใก | 045-123-4046 | 139.4994 | 35.52398 | 1548.0 |
9 | S14050 | ้ฟไน ๅ่ฅฟๅบ | 14 | ็ฅๅฅๅท็ | ็ฅๅฅๅท็ๆจชๆตๅธ็ฌ่ฐทๅบ้ฟไน ๅ่ฅฟไธไธ็ฎ | ใซใใฌใฏใฑใณใจใณใใใทใปใคใฏใขใฏใฏใใทใคใใใงใฆใก | 045-123-4053 | 139.4961 | 35.45918 | 1830.0 |
11 | S13052 | ๆฃฎ้ๅบ | 13 | ๆฑไบฌ้ฝ | ๆฑไบฌ้ฝ็บ็ฐๅธๆฃฎ้ไธไธ็ฎ | ใใฆใญใงใฆใใใใใทใขใชใใตใณใใงใฆใก | 042-123-4030 | 139.4383 | 35.55293 | 1087.0 |
12 | S14028 | ไบใๆฉๅบ | 14 | ็ฅๅฅๅท็ | ็ฅๅฅๅท็ๆจชๆตๅธ็ฌ่ฐทๅบไบใๆฉ็บ | ใซใใฌใฏใฑใณใจใณใใใทใปใคใฏใใฟใใใทใใงใฆ | 045-123-4042 | 139.4963 | 35.46304 | 1574.0 |
16 | S14012 | ๆฌ็งๅ็ฐๅบ | 14 | ็ฅๅฅๅท็ | ็ฅๅฅๅท็ๆจชๆตๅธไธญๅบๆฌ็งๅ็ฐ | ใซใใฌใฏใฑใณใจใณใใใทใใซใฏใใณใขใฏใฏใ | 045-123-4034 | 139.6582 | 35.42156 | 1341.0 |
18 | S14046 | ๅๅฑฑ็ฐๅบ | 14 | ็ฅๅฅๅท็ | ็ฅๅฅๅท็ๆจชๆตๅธ้ฝ็ญๅบๅๅฑฑ็ฐไธไธ็ฎ | ใซใใฌใฏใฑใณใจใณใใใทใใ ใญใฏใญใฟใคใใฟใคใใใงใฆใก | 045-123-4049 | 139.5916 | 35.56189 | 831.0 |
19 | S14022 | ้ๅญๅบ | 14 | ็ฅๅฅๅท็ | ็ฅๅฅๅท็้ๅญๅธ้ๅญไธไธ็ฎ | ใซใใฌใฏใฑใณใบใทใทใบใทใคใใใงใฆใก | 046-123-4036 | 139.5789 | 35.29642 | 1838.0 |
20 | S14011 | ๆฅๅๆฌ็บๅบ | 14 | ็ฅๅฅๅท็ | ็ฅๅฅๅท็ๆจชๆตๅธๆธฏๅๅบๆฅๅๆฌ็บๅไธ็ฎ | ใซใใฌใฏใฑใณใจใณใใใทใณใฆใใฏใฏใใจใทใใณใใงใฆใจใณใใงใฆใก | 045-123-4033 | 139.6316 | 35.54655 | 890.0 |
21 | S13016 | ๅฐ้ไบๅบ | 13 | ๆฑไบฌ้ฝ | ๆฑไบฌ้ฝๅฐ้ไบๅธๆฌ็บไธไธ็ฎ | ใใฆใญใงใฆใใณใฌใใคใทใใณใใงใฆใคใใใงใฆใก | 042-123-4015 | 139.5094 | 35.70018 | 1399.0 |
22 | S14034 | ๅทๅด้ๅทๅบ | 14 | ็ฅๅฅๅท็ | ็ฅๅฅๅท็ๅทๅดๅธๅฎฎๅๅบ้ๅท | ใซใใฌใฏใฑใณใซใฏใตใญใทใใคใใจใฏใใฌใฏ | 044-123-4044 | 139.5998 | 35.57693 | 1318.0 |
26 | S14048 | ไธญๅทไธญๅคฎๅบ | 14 | ็ฅๅฅๅท็ | ็ฅๅฅๅท็ๆจชๆตๅธ้ฝ็ญๅบไธญๅทไธญๅคฎไบไธ็ฎ | ใซใใฌใฏใฑใณใจใณใใใทใใ ใญใฏใใซใฌใฏใใฅใฆใชใฆใใใงใฆใก | 045-123-4051 | 139.5758 | 35.54912 | 1657.0 |
27 | S12007 | ไฝๅๅบ | 12 | ๅ่็ | ๅ่็ไฝๅๅธไธๅฟๆดฅ | ใใใฑใณใตใฏใฉใทใซใใทใ | 043-123-4001 | 140.1452 | 35.71872 | 1895.0 |
28 | S14026 | ่พปๅ ่ฅฟๆตทๅฒธๅบ | 14 | ็ฅๅฅๅท็ | ็ฅๅฅๅท็่คๆฒขๅธ่พปๅ ่ฅฟๆตทๅฒธไบไธ็ฎ | ใซใใฌใฏใฑใณใใธใตใฏใทใใธใใฆใใทใซใคใฌใณใใใงใฆใก | 046-123-4040 | 139.4466 | 35.32464 | 1732.0 |
29 | S13041 | ๅ ซ็ๅญๅบ | 13 | ๆฑไบฌ้ฝ | ๆฑไบฌ้ฝๅ ซ็ๅญๅธๅคงๅก | ใใฆใญใงใฆใใใใชใฆใธใทใชใชใใซ | 042-123-4026 | 139.4235 | 35.63787 | 810.0 |
31 | S14049 | ๅทๅดๅคงๅธซๅบ | 14 | ็ฅๅฅๅท็ | ็ฅๅฅๅท็ๅทๅดๅธๅทๅดๅบไธญ็ฌไธไธ็ฎ | ใซใใฌใฏใฑใณใซใฏใตใญใทใซใฏใตใญใฏใใซใผใตใณใใงใฆใก | 044-123-4052 | 139.7327 | 35.53759 | 962.0 |
32 | S14023 | ๅทๅดๅบ | 14 | ็ฅๅฅๅท็ | ็ฅๅฅๅท็ๅทๅดๅธๅทๅดๅบๆฌ็บไบไธ็ฎ | ใซใใฌใฏใฑใณใซใฏใตใญใทใซใฏใตใญใฏใใณใใงใฆใใใงใฆใก | 044-123-4037 | 139.7028 | 35.53599 | 1804.0 |
33 | S13018 | ๆธ ็ฌๅบ | 13 | ๆฑไบฌ้ฝ | ๆฑไบฌ้ฝๆธ ็ฌๅธๆพๅฑฑไธไธ็ฎ | ใใฆใญใงใฆใใญใจใปใทใใใคใใคใใใงใฆใก | 042-123-4017 | 139.5178 | 35.76885 | 1220.0 |
35 | S14027 | ๅ่คๆฒขๅบ | 14 | ็ฅๅฅๅท็ | ็ฅๅฅๅท็่คๆฒขๅธๅ่คๆฒข | ใซใใฌใฏใฑใณใใธใตใฏใทใใใใใธใตใฏ | 046-123-4041 | 139.4896 | 35.33762 | 1521.0 |
36 | S14021 | ไผๅขๅๅบ | 14 | ็ฅๅฅๅท็ | ็ฅๅฅๅท็ไผๅขๅๅธไผๅขๅๅไธ็ฎ | ใซใใฌใฏใฑใณใคใปใใฉใทใคใปใใฉใจใณใใงใฆใก | 046-123-4035 | 139.3129 | 35.40169 | 962.0 |
37 | S14047 | ็ธๆจกๅๅบ | 14 | ็ฅๅฅๅท็ | ็ฅๅฅๅท็็ธๆจกๅๅธๅไปฃ็ฐๅ ญไธ็ฎ | ใซใใฌใฏใฑใณใตใฌใใใฉใทใใจใใญใฏใใงใฆใก | 042-123-4050 | 139.3748 | 35.55959 | 1047.0 |
38 | S12013 | ็ฟๅฟ้ๅบ | 12 | ๅ่็ | ๅ่็็ฟๅฟ้ๅธ่ๅไธไธ็ฎ | ใใใฑใณใใฉใทใใทใทใใพใใคใใใงใฆใก | 047-123-4002 | 140.0220 | 35.66122 | 808.0 |
40 | S14042 | ๆฐๅฑฑไธๅบ | 14 | ็ฅๅฅๅท็ | ็ฅๅฅๅท็ๆจชๆตๅธไธญๅบๆฐๅฑฑไธไบไธ็ฎ | ใซใใฌใฏใฑใณใจใณใใใทใใซใฏใทใณใคใใทใฟใใใงใฆใก | 045-123-4047 | 139.6593 | 35.43894 | 1044.0 |
42 | S12030 | ๅ ซๅนกๅบ | 12 | ๅ่็ | ๅ่็ๅธๅทๅธๅ ซๅนกไธไธ็ฎ | ใใใฑใณใคใใซใฏใทใคใฏใฟใตใณใใงใฆใก | 047-123-4005 | 139.9240 | 35.72318 | 1162.0 |
44 | S14025 | ๅคงๅๅบ | 14 | ็ฅๅฅๅท็ | ็ฅๅฅๅท็ๅคงๅๅธไธๅ็ฐ | ใซใใฌใฏใฑใณใคใใใทใทใขใฏใ | 046-123-4039 | 139.4680 | 35.43414 | 1011.0 |
45 | S14045 | ๅๆจๅบ | 14 | ็ฅๅฅๅท็ | ็ฅๅฅๅท็ๅๆจๅธไธญ็บไบไธ็ฎ | ใซใใฌใฏใฑใณใขใใฎใทใใซใใงใฆใใใงใฆใก | 046-123-4048 | 139.3651 | 35.44182 | 980.0 |
47 | S12029 | ๆฑ้ๅบ | 12 | ๅ่็ | ๅ่็ๆตฆๅฎๅธๆฑ้ไธไธ็ฎ | ใใใฑใณใฆใฉใคในใทใใฌใทใใคใใใงใฆใก | 047-123-4004 | 139.8968 | 35.65086 | 1101.0 |
49 | S12053 | ้ซๆดฒๅบ | 12 | ๅ่็ | ๅ่็ๆตฆๅฎๅธ้ซๆดฒไบไธ็ฎ | ใใใฑใณใฆใฉใคในใทใฟใซในใดใใงใฆใก | 047-123-4006 | 139.9176 | 35.63755 | 1555.0 |
51 | S14024 | ไธ็ฐๅบ | 14 | ็ฅๅฅๅท็ | ็ฅๅฅๅท็ๅทๅดๅธๅคๆฉๅบไธ็ฐๅไธ็ฎ | ใซใใฌใฏใฑใณใซใฏใตใญใทใฟใใฏใใฟใจใณใใงใฆใก | 044-123-4038 | 139.5424 | 35.60770 | 972.0 |
52 | S14006 | ่ใ่ฐทๅบ | 14 | ็ฅๅฅๅท็ | ็ฅๅฅๅท็ๆจชๆตๅธ้ฝ็ญๅบ่ใ่ฐท | ใซใใฌใฏใฑใณใจใณใใใทใใ ใญใฏใฏใบใฌใค | 045-123-4031 | 139.5633 | 35.53573 | 1886.0 |
Commentary :
This code uses the Pandas library in Python to filter rows from a DataFrame nameddf_store
. The filter is based on a condition specified in thequery()
method, which takes a string argument representing a Boolean expression.tel_no
: This is assumed to be a column in thedf_store
DataFrame..
: This is the dot notation used to access a method or attribute of an object in Python.str
: This refers to the string methods of thetel_no
column.contains()
: This is a string method that checks whether a given substring or regular expression is present in each element of the column.r'^[0-9]{3}-[0-9]{3}-[0-9]{4}$'
: This is a regular expression pattern that matches strings that have the format of a North American phone number, which consists of three digits, a hyphen, three more digits, another hyphen, and four more digits. Ther
prefix before the pattern indicates that it is a raw string, which means that backslashes are treated as literal backslashes rather than escape characters. The curly braces{}
indicate that the preceding pattern should be repeated a specified number of times, and the$
at the end signifies the end of the string.
Thequery()
method is also specifying anengine
argument with the value'python'
. This is because the default engine used by Pandas isnumexpr
, which can be faster for some types of queries but may not support all types of regular expressions. Using the'python'
engine ensures that the regular expression is handled by the Python regex module.
The resulting filter will keep only those rows from thedf_store
DataFrame that have a valid North American phone number in thetel_no
column.
P-017: Sort customer data (df_customer) by birth_day(birth_day) in order of age and display all 10 items from the top.
df_customer.sort_values('birth_day').head(10)
customer_id | customer_name | gender_cd | gender | birth_day | age | postal_cd | address | application_store_cd | application_date | status_cd | |
---|---|---|---|---|---|---|---|---|---|---|---|
18817 | CS003813000014 | ๆๅฑฑ ่ใ ็พ | 1 | ๅฅณๆง | 1928-11-26 | 90 | 182-0007 | ๆฑไบฌ้ฝ่ชฟๅธๅธ่้ๅฐ********** | S13003 | 20160214 | 0-00000000-0 |
12328 | CS026813000004 | ๅๆ ๆ้ฝ | 1 | ๅฅณๆง | 1928-12-14 | 90 | 251-0043 | ็ฅๅฅๅท็่คๆฒขๅธ่พปๅ ๅ ็บ********** | S14026 | 20150723 | 0-00000000-0 |
15682 | CS018811000003 | ็ๆฒข ็พ้ | 1 | ๅฅณๆง | 1929-01-07 | 90 | 204-0004 | ๆฑไบฌ้ฝๆธ ็ฌๅธ้ๅกฉ********** | S13018 | 20150403 | 0-00000000-0 |
15302 | CS027803000004 | ๅ ๆ ๆ้ | 0 | ็ทๆง | 1929-01-12 | 90 | 251-0031 | ็ฅๅฅๅท็่คๆฒขๅธ้ต ๆฒผ่คใ่ฐท********** | S14027 | 20151227 | 0-00000000-0 |
1681 | CS013801000003 | ๅคฉ้ ๆ้ | 0 | ็ทๆง | 1929-01-15 | 90 | 274-0824 | ๅ่็่นๆฉๅธๅๅๆฑ********** | S12013 | 20160120 | 0-00000000-0 |
7511 | CS001814000022 | ้ถด็ฐ ้็ฉ | 1 | ๅฅณๆง | 1929-01-28 | 90 | 144-0045 | ๆฑไบฌ้ฝๅคง็ฐๅบๅๅ ญ้ท********** | S13001 | 20161012 | A-20090415-7 |
2378 | CS016815000002 | ๅฑฑๅ ็พ็ด | 1 | ๅฅณๆง | 1929-02-22 | 90 | 184-0005 | ๆฑไบฌ้ฝๅฐ้ไบๅธๆก็บ********** | S13016 | 20150629 | C-20090923-C |
4680 | CS009815000003 | ไธญ็ฐ ้็ฉ | 1 | ๅฅณๆง | 1929-04-08 | 89 | 154-0014 | ๆฑไบฌ้ฝไธ็ฐ่ฐทๅบๆฐ็บ********** | S13009 | 20150421 | D-20091021-E |
16070 | CS005813000015 | ้่ฐท ๆตๆขจ้ฆ | 1 | ๅฅณๆง | 1929-04-09 | 89 | 165-0032 | ๆฑไบฌ้ฝไธญ้ๅบ้ทบๅฎฎ********** | S13005 | 20150506 | 0-00000000-0 |
6305 | CS012813000013 | ๅฎ้ ๅๆ | 1 | ๅฅณๆง | 1929-04-09 | 89 | 231-0806 | ็ฅๅฅๅท็ๆจชๆตๅธไธญๅบๆฌ็ง็บ********** | S14012 | 20150712 | 0-00000000-0 |
Commentary :
This code uses the Pandas library in Python to sort rows of a DataFrame nameddf_customer
based on the values in thebirth_day
column in ascending order. The resulting sorted DataFrame is then limited to the first 10 rows using thehead()
method.df_customer
: This is the name of the DataFrame that is being sorted.sort_values()
: This is a Pandas method that is used to sort a DataFrame based on the values in one or more columns. In this case, thebirth_day
column is specified as the sort key.'birth_day'
: This is the name of the column that is used as the sort key..head(10)
: This is a method call that limits the output to the first 10 rows of the sorted DataFrame.
The resulting output will be the first 10 rows of thedf_customer
DataFrame, sorted in ascending order based on the values in thebirth_day
column. This will allow you to see the oldest 10 customers in the dataset, assuming thatbirth_day
represents the customers' birth dates.
P-018: Sort customer data (df_customer) by birth_day(birth_day) in descending order, displaying all 10 items from the top.
df_customer.sort_values('birth_day', ascending=False).head(10)
customer_id | customer_name | gender_cd | gender | birth_day | age | postal_cd | address | application_store_cd | application_date | status_cd | |
---|---|---|---|---|---|---|---|---|---|---|---|
15639 | CS035114000004 | ๅคงๆ ็พ้ | 1 | ๅฅณๆง | 2007-11-25 | 11 | 156-0053 | ๆฑไบฌ้ฝไธ็ฐ่ฐทๅบๆก********** | S13035 | 20150619 | 6-20091205-6 |
7468 | CS022103000002 | ็ฆๅฑฑ ใฏใใ | 9 | ไธๆ | 2007-10-02 | 11 | 249-0006 | ็ฅๅฅๅท็้ๅญๅธ้ๅญ********** | S14022 | 20160909 | 0-00000000-0 |
10745 | CS002113000009 | ๆด็ฐ ็ๆ ๅญ | 1 | ๅฅณๆง | 2007-09-17 | 11 | 184-0014 | ๆฑไบฌ้ฝๅฐ้ไบๅธ่ฒซไบๅ็บ********** | S13002 | 20160304 | 0-00000000-0 |
19811 | CS004115000014 | ๆพไบ ไบฌๅญ | 1 | ๅฅณๆง | 2007-08-09 | 11 | 165-0031 | ๆฑไบฌ้ฝไธญ้ๅบไธ้ทบๅฎฎ********** | S13004 | 20161120 | 1-20081231-1 |
7039 | CS002114000010 | ๅฑฑๅ ้ฅ | 1 | ๅฅณๆง | 2007-06-03 | 11 | 184-0015 | ๆฑไบฌ้ฝๅฐ้ไบๅธ่ฒซไบๅ็บ********** | S13002 | 20160920 | 6-20100510-1 |
3670 | CS025115000002 | ๅฐๆณ ๅคๅธ | 1 | ๅฅณๆง | 2007-04-18 | 11 | 245-0018 | ็ฅๅฅๅท็ๆจชๆตๅธๆณๅบไธ้ฃฏ็ฐ็บ********** | S14025 | 20160116 | D-20100913-D |
12493 | CS002113000025 | ๅบๆซ ใพใชใฟ | 1 | ๅฅณๆง | 2007-03-30 | 12 | 184-0015 | ๆฑไบฌ้ฝๅฐ้ไบๅธ่ฒซไบๅ็บ********** | S13002 | 20171030 | 0-00000000-0 |
15977 | CS033112000003 | ้ท้ ็พ็ด | 1 | ๅฅณๆง | 2007-03-22 | 12 | 245-0051 | ็ฅๅฅๅท็ๆจชๆตๅธๆธๅกๅบๅ็ฌ็บ********** | S14033 | 20150606 | 0-00000000-0 |
5716 | CS007115000006 | ็ฆๅฒก ็ฌ | 1 | ๅฅณๆง | 2007-03-10 | 12 | 285-0845 | ๅ่็ไฝๅๅธ่ฅฟๅฟๆดฅ********** | S12007 | 20151118 | F-20101016-F |
15097 | CS014113000008 | ็ขๅฃ ่็ท | 1 | ๅฅณๆง | 2007-03-05 | 12 | 260-0041 | ๅ่็ๅ่ๅธไธญๅคฎๅบๆฑๅ่********** | S12014 | 20150622 | 3-20091108-6 |
Commentary :
This code uses the Pandas library in Python to sort rows of a DataFrame nameddf_customer
based on the values in thebirth_day
column in descending order. The resulting sorted DataFrame is then limited to the first 10 rows using thehead()
method.df_customer
: This is the name of the DataFrame that is being sorted.sort_values()
: This is a Pandas method that is used to sort a DataFrame based on the values in one or more columns. In this case, thebirth_day
column is specified as the sort key.'birth_day'
: This is the name of the column that is used as the sort key.ascending=False
: This argument is used to specify that the sort order should be in descending order rather than the default ascending order..head(10)
: This is a method call that limits the output to the first 10 rows of the sorted DataFrame.
The resulting output will be the first 10 rows of thedf_customer
DataFrame, sorted in descending order based on the values in thebirth_day
column. This will allow you to see the youngest 10 customers in the dataset, assuming thatbirth_day
represents the customers' birth dates.
P-019: Rank the receipt details data (df_receipt) in descending order of sales amount per item (amount) and display 10 items from the top. The items shall display the customer ID (customer_id), sales amount (amount) and the rank assigned. If the sales amount (amount) is equal, the same rank shall be assigned.
df_tmp = pd.concat([df_receipt[['customer_id', 'amount']]
,df_receipt['amount'].rank(method='min',
ascending=False)], axis=1)
df_tmp.columns = ['customer_id', 'amount', 'ranking']
df_tmp.sort_values('ranking').head(10)
customer_id | amount | ranking | |
---|---|---|---|
1202 | CS011415000006 | 10925 | 1.0 |
62317 | ZZ000000000000 | 6800 | 2.0 |
54095 | CS028605000002 | 5780 | 3.0 |
4632 | CS015515000034 | 5480 | 4.0 |
72747 | ZZ000000000000 | 5480 | 4.0 |
10320 | ZZ000000000000 | 5480 | 4.0 |
97294 | CS021515000089 | 5440 | 7.0 |
28304 | ZZ000000000000 | 5440 | 7.0 |
92246 | CS009415000038 | 5280 | 9.0 |
68553 | CS040415000200 | 5280 | 9.0 |
Commentary :
The given code is performing the following operations:
Concatenating two dataframesdf_receipt[['customer_id', 'amount']]
anddf_receipt['amount'].rank(method='min', ascending=False)
along the columns axis (axis=1) using thepd.concat()
function. The resulting dataframe is assigned to the variabledf_tmp
. The first dataframedf_receipt[['customer_id', 'amount']]
contains two columns 'customer_id' and 'amount' from a larger dataframedf_receipt
, whereas the second dataframe is the rank of the 'amount' column calculated using the 'min' method in descending order.
Renaming the columns of the concatenated dataframedf_tmp
to 'customer_id', 'amount', and 'ranking' using thedf_tmp.columns
attribute.
Sorting thedf_tmp
dataframe by the 'ranking' column in ascending order using thesort_values()
method and returning the top 10 rows using thehead(10)
method.
Overall, the code is concatenating the 'customer_id' and 'amount' columns from thedf_receipt
dataframe with the rank of 'amount' calculated using the 'min' method in descending order. The resulting dataframe is sorted by the 'ranking' column and returns the top 10 rows with the highest rank, which represents the customers who spent the most on their purchases.
P-020: For receipt details data (df_receipt), assign ranks in descending order of sales amount (amount) per item and display the first 10 items. Items shall display the customer ID (customer_id), sales amount (amount) and the rank assigned. Even if the sales amount (amount) is equal, a different rank should be assigned.
df_tmp = pd.concat([df_receipt[['customer_id', 'amount']]
,df_receipt['amount'].rank(method='first',
ascending=False)], axis=1)
df_tmp.columns = ['customer_id', 'amount', 'ranking']
df_tmp.sort_values('ranking').head(10)
customer_id | amount | ranking | |
---|---|---|---|
1202 | CS011415000006 | 10925 | 1.0 |
62317 | ZZ000000000000 | 6800 | 2.0 |
54095 | CS028605000002 | 5780 | 3.0 |
4632 | CS015515000034 | 5480 | 4.0 |
10320 | ZZ000000000000 | 5480 | 5.0 |
72747 | ZZ000000000000 | 5480 | 6.0 |
28304 | ZZ000000000000 | 5440 | 7.0 |
97294 | CS021515000089 | 5440 | 8.0 |
596 | CS015515000083 | 5280 | 9.0 |
11275 | CS017414000114 | 5280 | 10.0 |
Commentary :
The given code is performing the following operations:
Concatenating two dataframesdf_receipt[['customer_id', 'amount']]
anddf_receipt['amount'].rank(method='first', ascending=False)
along the columns axis (axis=1) using thepd.concat()
function. The resulting dataframe is assigned to the variabledf_tmp
. The first dataframedf_receipt[['customer_id', 'amount']]
contains two columns 'customer_id' and 'amount' from a larger dataframedf_receipt
, whereas the second dataframe is the rank of the 'amount' column calculated using the 'first' method in descending order.
Renaming the columns of the concatenated dataframedf_tmp
to 'customer_id', 'amount', and 'ranking' using thedf_tmp.columns
attribute.
Sorting thedf_tmp
dataframe by the 'ranking' column in ascending order using thesort_values()
method and returning the top 10 rows using thehead(10)
method.
Overall, the code is concatenating the 'customer_id' and 'amount' columns from thedf_receipt
dataframe with the rank of 'amount' calculated using the 'first' method in descending order. The resulting dataframe is sorted by the 'ranking' column and returns the top 10 rows with the highest rank, which represents the customers who spent the most on their purchases. The only difference between this code and the previous one is the rank method used. The 'first' method is used here, which assigns the same rank to the equal values of 'amount' column whereas the 'min' method assigns the lowest rank to the equal values of 'amount' column.
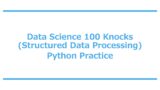
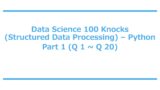
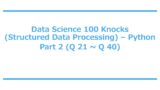
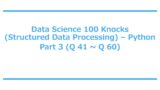
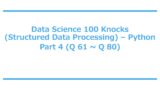
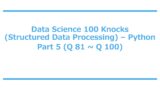
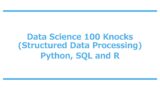
Comment